Department of Statistics
2012 Seminars
Seminars by year: Current | 2004 | 2005 | 2006 | 2007 | 2008 | 2009 | 2010 | 2011 | 2012

Speaker: Prof. Jari Kaipio
Affiliation:
When: Thursday, 13 December 2012, 4:00 pm to 5:00 pm
Where: 303.B09 Science Centre
We discuss Bayesian modelling of errors that are induced by model uncertainties and practical implementational constraints. Our focus is on problems that are severely resource limited with respect to computational power, memory and time. This is the common case in industrial and biomedical problems in general, and often also in other problems, in which a continuous stream of data is to be processed. Such problems suggest to employ highly approximative reduced order models, and often only some of the actual unknowns are of interest.
Such models provide always a more or less simplified approximation for the physical reality.
http://www.math.auckland.ac.nz/Directory/jahia/staff-profile-jahia.php?upi=jkai005
Construction of irregular histograms by penalized maximum likelihood: new solutions to an old problem
Speaker: Dr. Ciprian Giurcaneanu
Affiliation: U. Auckland
When: Tuesday, 20 November 2012, 10:00 am to 11:00 am
Where: 303-B07
Theoretical advances of the last decade have led to novel methodologies for probability density estimation by irregular histograms and penalized maximum likelihood. In this talk we will consider two of them: the first one is based on the idea of minimizing the excess risk, while the second one employs the concept of the normalized maximum likelihood (NML). Apparently, the previous literature does not contain any comparison of the two approaches. This motivates us to provide theoretical and empirical results for clarifying the relationship between the two methodologies. Additionally, a new variant of the NML histogram will be introduced. For the sake of completeness, we will consider in our comparisons a more advanced NML-based method that uses the measurements to approximate the unknown density by a mixture of densities selected from a predefined family. We will also discuss briefly some new findings in connection with the performance of histogram-based entropy estimators.
The content of the talk is based on novel results obtained by the author and his collaborators from the University of Helsinki (Finland): MSc P. Luosto and Dr. P. Kontkanen.
http://www.cs.auckland.ac.nz/~cgiu216/
An adaptive resampling test for detecting the presence of significant predictors
Speaker: Prof. Ian McKeague
Affiliation: Columbia U.
When: Monday, 19 November 2012, 11:00 am to 12:00 pm
Where: 303-B09
This talk discusses a new screening procedure based on marginal linear regression for detecting the presence of a significant predictor. Standard inferential methods are known to fail in this setting due to the non-regular limiting behavior of the estimated regression coefficient of the selected predictor; in particular, the limiting distribution is discontinuous at zero as a function of the regression coefficient of the predictor maximally correlated with the outcome. To circumvent this non-regularity, we propose a bootstrap procedure based a local model in order to better reflect small-sample behavior at a root-n scale in the neighborhood of zero.
The proposed test is adaptive in the sense that it employs thresholding to distinguish situations in which a centered percentile bootstrap applies, and otherwise adapts to the local asymptotic behavior of the test statistic in a way that depends continuously on the local parameter. The performance of the approach is evaluated using a simulation study, and applied to an example involving gene expression data.
The talk is based on joint work with Min Qian.
http://www.columbia.edu/~im2131
hwriterPlus: A Simple Approach to Reproducible Research
Speaker: Assoc. Prof. David Scott
Affiliation: U. Auckland
When: Thursday, 8 November 2012, 4:00 pm to 5:00 pm
Where: ECE briefing room 303.257
There are now many approaches to reproducible research or automatic report generation using R. Besides Sweave which is probably the best known, other software includes odfWeave, SWord, org-babel, knitr, R2HTML and hwriter.
I have enhanced hwriter in my package hwriterPlus, which will produce html, and somewhat incidentally, Microsoft Word documents. I will describe the package and give examples.
http://www.stat.auckland.ac.nz/~dscott/
The gaps left by a Brownian motion
Speaker: Dr. Jesse Goodman
Affiliation: Leiden U.
When: Thursday, 11 October 2012, 4:00 pm to 5:00 pm
Where: 303.B09 Science Centre
Run a Brownian motion - a continuous, symmetrical, Gaussian random motion - on a torus for a long time. How large are the random gaps left behind when the path is removed?
In three (or more) dimensions, we find that there is a deterministic spatial scale common to all the large gaps anywhere in the torus. Moreover, we can identify whether a gap of a given shape is likely to exist on this scale, in terms of a single parameter, the classical (Newtonian) capacity. I will describe why this allows us to identify a well-defined "component" structure in our random porous set.
http://www.math.leidenuniv.nl/~goodmanja/
"Detecting carryover effects in prevention trials" and " P-Spline Vector Generalized Additive Models"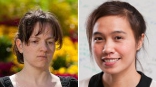
Speaker: Gwynn Sturdevant and Chanatda Somchit
Affiliation: U. Auckland
When: Thursday, 20 September 2012, 4:00 pm to 5:00 pm
Where: ECE briefing room 257
First up is Gwynn. The TROPHY study in 2006 claimed that a blood pressure drug still kept working two years after people stopped taking it. My research looks at why this was wrong, whether the trial design can be changed so that the problems don't arise (no, it can't), and, in the rest of my PhD, ways to analyse the data to remove the problems.
Chanatda will discuss generalizing the use of penalized regression smoothers, based on P-splines for GAM modeling to VGLM/VGAM classes. She will also talk about integrating a stable and efficient smoothing parameter selection methodology in the framework of vector generalized additive models (VGAMs) using well founded criteria such as generalized cross-validation (GCV) or the Akaike information criterion (AIC).
Please note that there will be no food and drink directly after the seminar. However you are welcome to join us and our students for nibbles and drinks at 6pm in the Commerce A common room (Building 114, Room 118)
Building clinically useful molecular diagnostics - or - Taming the three headed dragon: Biology, Statistics, and MarketingSpeaker: Nicholas Knowlton
Affiliation: U. Auckland
When: Thursday, 13 September 2012, 4:00 pm to 5:00 pm
Where: ECE briefing room 257
Every year hundreds of molecular diagnostics are presented in the literature; however, almost none make it into a clinician's toolbox. Why? Taking a clinical diagnostic to market is a delicate dance between clinicians, statisticians and marketers. This seminar will discuss several ways to integrate biology and marketing into the model building process to assist with patent potential and regulatory review. These techniques will be discussed in the context of a recently launched molecular diagnostic for Rheumatoid Arthritis. Additionally, techniques that dissect canned models for additional biology will be discussed in the context of breast cancer.
Regulating arrivals to a queueSpeaker: Prof. Moshe Haviv
Affiliation: The Hebrew U. of Jerusalem
When: Thursday, 9 August 2012, 4:00 pm to 5:00 pm
Where: ECE briefing room 257
The talk will first address the issue of negative externalities in queues: One who decides to join a queue ignores the extra waiting cost one inflicts by joining on others. Had these externalities being considered by individuals, the rate of joining the queue would have been dropped considerably. We will look at various regulation schemes.
Under such schemes different rules are imposed on the queue so as customers, while still selfishly minding only their own good, now behave in a socially optimal way.
The talk will address a few such schemes. The first is the imposition of entry fees, in particular, asking payments which are service-length based. Alternatively, extra holding costs can be imposed. The second is by bidding for a position in the queue.
Interestingly, the rate of opting out coincides with the socially optimal one if customers who decide to join are getting priority based on an entry fee which they themselves decide to pay. A third is by granting service not on a first-come first-served (FCFS) basis. Indeed, surprisingly, FCFS is the socially worst queueing regime. More than that, any service regime but FCFS leads to the socially desired joining rate if customers are allowed to renege. A fourth, is my letting customers know their service times in advance and letting them to decide if to join or not. Under the shortest job first (SJF) scheduling policy, this scheme leads to self regulation: Left for themselves, customers still behave in a socially optimal way.
http://pluto.huji.ac.il/~haviv/
Causal inference in observational settings. A preliminary review
Speaker: Prof. Peter Davis
Affiliation: U. Auckland
When: Thursday, 26 July 2012, 4:00 pm to 5:00 pm
Where: ECE briefing room 257
Most social science and public health research is carried out in observational settings. Yet such research can rarely generate inferences of a conventional causal status to inform theory and intervention. However, advances have been made in helping researchers develop and draw more credible inferences from such data. These advances have come particularly from logicians and philosophers, who have generalised to observational work a variant of the model of causal inference based on the experiment (potential outcomes, counterfactuals) and from applied statisticians, particularly those working in econometrics and in educational and applied social research who are concerned with drawing conclusions about policies and interventions. This seminar will review this work, which is preparatory to compiling a reader on the topic in the Sage Benchmark series in Social Research Methods. Examples will be given from the literature.
http://artsfaculty.auckland.ac.nz/staff/?UPI=pdav008&Name=Peter%20Davis
Regularly paved random histograms and their statistical applications
Speaker: Dr. Raazesh Sainudiin
Affiliation: U. Canterbury
When: Thursday, 12 July 2012, 4:00 pm to 5:00 pm
Where: ECE briefing room 257
We present a novel method for averaging a sequence of histogram states visited by a Metropolis-Hastings Markov chain whose stationary distribution is the posterior distribution over a dense space of tree-based histograms. The computational efficiency of our posterior mean histogram estimate relies on a statistical data-structure that is sufficient for nonparametric density estimation of massive, multi-dimensional metric data. This data-structure is formalized as statistical regular paving (SRP).
A regular paving (RP) is a binary tree obtained by selectively bisecting boxes along their first widest side. SRP augments RP by mutably caching the recursively computable sufficient statistics of the data. The base Markov chain used to propose moves for the Metropolis-Hastings chain is a random walk that data-adaptively prunes and grows the SRP histogram tree. We use a prior distribution based on Catalan numbers and initialize our Markov chain along a path visited by an asymptotically consistent randomized priority queue. The performance of our posterior mean SRP histogram is empirically assessed for large sample sizes (up to 100 million points) simulated from several high dimensional distributions.
The arithmetical operations over SRPs allow us to obtain marginal and conditional densities as well as highest 1-\alpha coverage regions. Natural applications include Approximate Bayesian Computations and visualization of high dimensional density estimates and their arithmetical expressions.
This is joint work with Jennifer Harlow, Dominic Lee and Gloria Teng.
http://www.math.canterbury.ac.nz/~r.sainudiin/
A Survey of Analysis and Simulation Techniques for Dynamic Microsimulation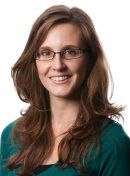
Speaker: Jessica Thomas
Affiliation: U. Auckland
When: Thursday, 14 June 2012, 4:00 pm to 5:00 pm
Where: 303-B11
The dynamic microsimulation approach uses individual-level longitudinal data to create virtual life histories. We have developed such a model of the early life-course. The model, embedded in a software tool, is designed to inform policy making by testing relevant scenarios. Key to the overall model are the rules that inform the transitions of children from year to year. These rules are typically derived from statistical models which need to meet the unique requirements of dynamic microsimulation. A number of analysis approaches were canvassed and an evaluation of these will be presented
[Jessica is a member of the Statistical Consulting Centre at The University of Auckland, through which she works mainly with COMPASS (Centre of Methods and Policy Application in the Social Sciences) on a large microsimulation project.]
http://www.stat.auckland.ac.nz/showperson?firstname=Jessica&surname=Thomas
The probability of extinction in a branching process and its relationship with moments of the offspring distribution
Speaker: Sterling Sawaya
Affiliation: U. Otago
When: Tuesday, 12 June 2012, 11:00 am to 12:00 pm
Where: 303-B11
We model the growth of a biological population over time using a Galton-Watson (discrete) branching process. The fitness of a population can be evaluated using several statistical approaches, with the log growth being the most popular. In addition to growth, the probability of extinction can be used to measure the long-term success of any population. Here, we will discuss the interplay between this probability and the moments of the offspring distribution. Using results from the field of decision theory we will show that the probability of extinction decreases with increasing odd moments and increases with increasing even moments, a property which is intuitively clear.
There is no closed form solution to calculate the probability of extinction, and numerical methods are often used to infer its value. Alternatively, one can use analytical approaches to generate bounds on the extinction probability. I will discuss these bounds, focusing on the theory of s-convex ordering of random variables, a method used in the field of actuarial sciences. This method utilizes the first few moments of the offspring distribution to generate "worst case scenario" distributions, which can then be used to find upper bounds on the probability of extinction. I will present these methods and discuss their merits in the field of population biology.
http://www.otago.ac.nz/crg/staff/students/otago023396.html
Applying statistics in fisheries science
Speaker: Dr. Ian Tuck
Affiliation: NIWA / U. Auckland
When: Wednesday, 6 June 2012, 11:00 am to 12:00 pm
Where: 303-B07
I have recently joined the department on a one day a week basis as part of the NIWA/UoA Joint Graduate School in Marine Science. I spend the other four days a week working at NIWA in Auckland, on fisheries.
I am taking the opportunity of this seminar to introduce myself to the department, talk a bit about my background, and describe some of the recent applications of statistical methods I have undertaken in the course of my fisheries research.
http://www.niwa.co.nz/key-contacts/ian-tuck
Optimal Designs for Stated Choice Experiments that Incorporate Position Effects
Speaker: Dr. Stephen Bush
Affiliation: U. T. Sydney
When: Thursday, 31 May 2012, 4:00 pm to 5:00 pm
Where: 303-B07
Choice experiments are widely used in transportation, marketing, health and environmental research to measure consumer preferences. From these consumer preferences, we can calculate willingness to pay for an improved product or state, and hence make policy decisions based on these preferences.
In a choice experiment, we present choice sets to the respondent sequentially. Each choice set consists of m options, each of which describes a product or state, which we generically call an item. Each item is described by a set of attributes, the features that we are interested in measuring. Respondents are asked to select the most preferred item in each choice set. We then use the multinomial logit model to determine the importance of each attribute.
In some situations we may be interested in whether an item's position within the choice set affects the probability that the item is selected. This problem is reminiscent of donkey voting in elections, and can also be seen in the design of tournaments, where the home team is expected to have an advantage.
In this presentation, we present a discussion of stated choice experiments, and then discuss a model that incorporates position effects for choice experiments with arbitrary m. This is an extension of the model proposed by Davidson and Beaver (1977) for m = 2. We give optimal designs for the estimation of attribute main effects plus the position effects under the null hypothesis of equal selection probabilities. We conclude with some simulations that compare how well optimal designs and near-optimal designs estimate the attribute main effects and position effects for various sets of parameter values.
http://datasearch2.uts.edu.au/science/staff/details.cfm?StaffId=4018
Linear regression modulo p, learning with errors, and applications to cryptography
Speaker: Assoc. Prof. Steven Galbraith
Affiliation: U. Auckland
When: Thursday, 24 May 2012, 4:00 pm to 5:00 pm
Where: ECE briefing room 257
Public key cryptography is a very active research area, with many theoretical and practical challenges.
One area of current theoretical interest is "lattice-based cryptography". Many of the computational problems in this area have a rather statistical flavour. In particular, I will discuss the learning with errors problem (LWE), which can be thought of as linear regression modulo a prime. LWE was introduced by Oded Regev in 2005 and has a number of cryptographic applications. The talk will be a survey of some of this work and will be accessible to a general audience.
http://www.math.auckland.ac.nz/~sgal018/
Do your data fit your phylogenetic tree?
Speaker: Dr Steffen Klaere
Affiliation: Department of Statistics, University of Auckland
When: Thursday, 10 May 2012, 4:00 pm to 5:00 pm
Where: ECE Seminar Room 303.257, Science Centre
Phylogenetic methods are used to infer ancestral relationships based on genetic and morphological sequences. What started as more sophisticated clustering has now become a more and more complex machinery of estimating ancestral processes and divergence times. One major branch of inference are maximum likelihood methods. Here, one selects the parameters from a given model class for which the data are more likely to occur than for any other set of parameters of the same model class. Most analysis of real data are executed using such methods.
However, one step of statistical inference that has little exposure to application is the goodness of fit test between parameters and the data. There seem to be various reasons for this behaviour, users are either content with using a bootstrap approach to obtain support for the inferred topology, are afraid that a goodness of fit test would find little or no support for their phylogeny thus demeaning their carefully assembled data, or they simply lack the statistical background to acknowledge this step.
Recently, methods to detect those sections of the data which do not support the inferred model have been proposed, and strategies to explain these differences have been devised. In this talk I will present and discuss some of these methods, their shortcomings and possible ways of improving them.
http://www.stat.auckland.ac.nz/showperson?firstname=Steffen&surname=Klaere
Problems for the clairvoyant demon
Speaker: Prof. Geoffrey Grimmett
Affiliation: U. Cambridge
When: Thursday, 3 May 2012, 2:00 pm to 3:00 pm
Where: Conference Centre Lecture Theatre, Room 423-342, 22 Symonds street
The 2012 Forder Colloquium
The clairvoyant demon can see into the future. But how does this help `it' to do its work?
I will describe three apparently simple problems for the demon involving infinite sequences of coin tosses.
Two of these problems were formulated by Peter Winkler. The third problem is provocative and unsolved. It asks whether one random sequence may be embedded within another. There are connections to earlier work by others on biLipschitz embeddings and quasi-isometries, and even to the Borsuk-Ulam theorem of topological combinatorics.
Geoffrey Grimmett is the Professor of Mathematical Statistics in the Statistical Laboratory, University of Cambridge. He is known for his work on the mathematics of random systems arising in probability theory and statistical mechanics, especially percolation theory and the contact process. Professor Grimmett is the 2012 Forder Lecturer and - in this quality - he is touring New Zealand mathematics departments, giving a series of departmental colloquia and public lectures.
http://www.statslab.cam.ac.uk/~grg/
Tree models for difference and change detection in a complex environment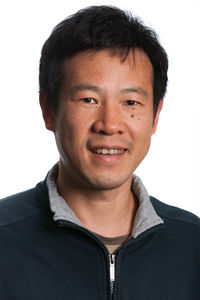
Speaker: Dr. Yong Wang
Affiliation: U. Auckland
When: Thursday, 26 April 2012, 4:00 pm to 5:00 pm
Where: ECE briefing room 257
In this talk, I will describe a new family of tree-structured models, which are called ``differential trees''. A differential tree model is constructed from multiple data sets and aims to detect distributional differences between them. The new methodology differs from the existing difference and change detection techniques in its nonparametric nature, model construction from multiple data sets, and applicability to high-dimensional data. Through a detailed study of an arson case in New Zealand, where an individual is known to have been laying vegetation fires within a certain time period, we illustrate how these models can help detect changes in the frequencies of event occurrences and uncover unusual clusters of events in a complex environment.
http://www.stat.auckland.ac.nz/showperson?firstname=Yong&surname=Wang
From trajectories to averages: an improved description of the heterogeneity of substitution rates along lineages.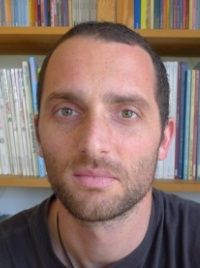
Speaker: Dr. Stephane Guindon
Affiliation: U. Auckland
When: Thursday, 19 April 2012, 4:00 pm to 5:00 pm
Where: ECE briefing room 257
The accuracy and precision of species divergence date estimation from molecular data strongly depend on the models describing the variations of substitution rates along a phylogeny. These models generally assume that rates randomly fluctuate along branches from one node to the next. However, for mathematical convenience, the stochasticity of such process is ignored when translating such rate trajectories into branch lengths.
The present study addresses this shortcoming. A new approach is described that explicitly considers the average substitution rates along branches as random quantities, resulting in a more realistic description of the variations of evolutionary rates along lineages. This method provides more precise estimates of the rate autocorrelation parameter as well as divergence times. Also, simulation results indicate that ignoring the stochastic variation of rates along edges can lead to significant overestimation of specific node ages.
Altogether, the new approach introduced here is a step forward to designing biologically relevant models of rate evolution that are well-suited to data sets with dense taxon sampling which are likely to present rate autocorrelation.
http://compevol.auckland.ac.nz/stephane-guindon/
Signal analysis by stochastic complexity
Speaker: Dr. Ciprian Giurcaneanu
Affiliation: Helsinki I.I.T.
When: Thursday, 19 April 2012, 11:00 am to 12:00 pm
Where: 303-B07
The talk will be focused on the applications of the stochastic complexity (SC), which has been introduced by Prof. Jorma Rissanen in the framework of model selection. During recent years, we have proposed various solutions based on SC for solving the following problems:
(1) Variable selection in Gaussian linear regression;
(2) AR order selection when the coefficients of the model are estimated with forgetting-factor least-squares algorithms;
(3) Estimation of the number of sine-waves in Gaussian noise when the sample size is small;
(4) Quantifying the dependence between time series, with applications to the EEG analysis in a mild epileptic paradigm;
(5) Composite hypothesis testing by optimally distinguishable distributions.
During the talk, we will provide a short overview of the results outlined above by emphasizing the superiority of SC in comparison with other methods.
http://www.cs.tut.fi/~cipriand/
Making peace with p's: Bayesian interpretations of two-sided tests
Speaker: Associate Prof. Ken Rice
Affiliation: U. Washington
When: Tuesday, 17 April 2012, 11:00 am to 12:00 pm
Where: ECE briefing room 257
Statistical testing has a long history of controversy; the Fisher and Neyman-Pearson approaches have fundamental differences, and neither of them agree with standard Bayesian procedures. In this talk, we set out an approach to testing that dissipates some of this controversy. Using decision theory, we develop tests as trade-offs, where the user balances potential inaccuracy of a point estimate against the `embarrassment' of making no scientific conclusion at all. The resulting Bayesian tests are simple, and their repeated-sampling properties can be determined straightforwardly. The same motivation also provides straightforward interpretations of two-sided p-values, calibrating them directly through scientifically-relevant quantities, rather than via statistical evaluation of Type I error rates. Time permitting, extensions to set-valued decisions, model-robust inference and shrinkage estimates may also be considered.
http://faculty.washington.edu/kenrice/
Bayesian nonparametrics and scalable probabilistic inference
Speaker: Jared Tobin
Affiliation: U. Auckland
When: Thursday, 12 April 2012, 4:00 pm to 5:00 pm
Where: ECE briefing room 257
Bayesian nonparametrics is a generalization of both parametric and frequentist statistics, characterized by the specification of Bayesian probability models on infinite-dimensional parameter spaces. Establishing an important class of models in statistics and machine learning, Bayesian nonparametrics combines the adaptive model complexity of nonparametric approaches with the probabilistic interpretation and inherent parsimony embedded in Bayesian techniques. These models have been successfully applied to problems in computer vision, natural language processing, finance, robot control, and genetics, amongst others.
Implementations of nonparametric Bayesian models are often criticized for being slow, requiring the inversion of large matrices or compute-intensive sampling for inference. The research community has identified a specific, consensus need for the development of better inference procedures to meet the needs of modern-day problems and scale nonparametric Bayesian models for widespread use.
This seminar will provide an overview of Bayesian nonparametrics, discussing some theory, applications, and cues towards performing inference at scale.
http://www.stat.auckland.ac.nz/showperson?firstname=Jared&surname=Tobin
Computationally Efficient Methods for Bayesian Inference / Bayesian Modeling of Trait Based Community Assembly.
Speaker: Dr. Chaitanya Joshi
Affiliation: U. Waikato
When: Thursday, 5 April 2012, 4:00 pm to 5:00 pm
Where: 303-B07
I plan to give two short talks to reflect the two main themes of my research.
Computationally Efficient Methods for Bayesian Inference:
Computational efficiency has become necessary in many applications of Bayesian inference. In this direction, attempts have been made to implement non-MCMC based approaches. One such approach is the `Integrated Nested Laplace Approximation' (INLA) developed by Rue et al. (2009). Inspired by the idea, we have developed a computationally efficient method to implement Bayesian Inference on stochastic differential equation (SDE) models. In addition to being computationally efficient, our approach is also easy to implement. In this talk, I'll give a brief overview of our approach and also talk about some future directions.
Bayesian Modeling of Trait Based Community Assembly:
Ecologists have long observed that phenotypic traits of species influence where they occur in the landscape but only few attempts at modeling this phenomenon have been made. Existing model MaxEnt ignores phenotypic trait variation within species. To evaluate the importance of intraspecific trait variation in community assembly we have developed Traitspace, a new mathematical framework that explicitly models the filtering of individual-level plant traits through the environment. We incorporate the full distribution of observed trait values. This approach allows species to overlap in trait space and allows individuals within species to differ. We use Traitspace to predict species relative abundance and also discuss the possible future developments of this model.
Response adaptive repeated measurement designs in the presence of treatment effects
Speaker: Prof. Keumhee Chough
Affiliation: U. Alberta
When: Thursday, 22 March 2012, 4:00 pm to 5:00 pm
Where: ECE briefing room 303.257, Science Centre
A multiple-objective allocation strategy was recently proposed for constructing response-adaptive repeated measurement designs for continuous responses. In this talk, we briefly review and extend the allocation strategy to constructing response-adaptive repeated measurement designs for binary responses. Through computer simulations, we find that the allocation strategy developed for continuous responses also works well for binary responses and it can successfully allocate more patients to better treatment sequences without sacrificing much of estimation precision. However, design efficiency in terms of mean squared error drops sharply as more emphasis is placed on increasing treatment benefit than estimation precision. We also find that the allocation for the binary response case is rather largely spread out over the treatment sequences considered, leading to designs with many treatment sequences, unlike the continuous response case, where the adaptive designs often coincided with the fixed optimal designs. I will also briefly introduce optimal N of 1 trial designs.
http://www.math.ualberta.ca/~kcarrier/
Delights of directional statistics: (a) free-lunch learning, (b) crystals, earthquakes and orthogonal axial frames
Speaker: Professor Peter Jupp
Affiliation: U. St. Andrews
When: Thursday, 15 March 2012, 4:00 pm to 5:00 pm
Where: ECE briefing room 303.257, Science Centre
Observations that are directions, axes, or rotations require the techniques of directional statistics. This talk aims to illustrate the special flavour of this area through glimpses at two topics.
(a) Free-lunch learning
Free-lunch learning (FLL) is a phenomenon in which relearning partially-forgotten mental associations induces recovery of other associations. When memory is modelled in terms of an artificial neural network, the extent of FLL can be quantified in geometrical terms and involves Grassmann manifolds of subspaces of the weight space. Joint work with Jim Stone (Psychology, Sheffield) will be described, in which simple properties of uniform distributions yield results on the expected amount of FLL. The form of forgetting plays an important role.
(b) Crystals, earthquakes and orthogonal axial frames
Orthogonal axial frames are (ordered) sets of orthogonal axes. They arise as (i) key geometrical elements (known in seismology as `focal mechanisms') of earthquakes, (ii) principal axes of certain physical tensors (e.g. stress tensors), (iii) axes of orthorhombic crystals. Some tools for the analysis of data that are orthogonal axial frames will be will be described. This is joint work with Richard Arnold (Wellington).
http://www.mcs.st-and.ac.uk/~pej/
Optimal Asset Pricing
Speaker: Dr. Rolf Turner
Affiliation: Department of Statistics, University of Auckland
When: Thursday, 8 March 2012, 4:00 pm to 5:00 pm
Where: ECE briefing room 303.257, Science Centre
It is a well-known phenomenon that airline passengers travelling on the same flight (same origin and same destination) and in the same class (cabin) will often have paid substantially different fares. This apparent anomaly in the pricing pattern is due to the fact there is a time-varying elasticity of demand (or "price sensitivity") for this particular "product".
My co-author Pradeep Banerjee and I have developed a differential equations model which permits one to derive an optimal pricing policy in such a setting. (The policy is "optimal" in terms of the expected value of a stock of goods at a specified time.) Deriving the optimal policy requires a model for the price sensitivity and for an inhomogeneous Poisson arrival rate of customers. So far we have worked with smooth price sensitivity functions. However it is somewhat easier to translate intuitive conjectures about price sensitivity into a function framed as being piecewise linear in price.
In this talk I will explain a bit about how the differential equations for the optimal prices are derived, and then discuss how the technique must be adjusted to deal with the piecewise linear setting. I will also discuss some of the techniques that I and my Summer Scholarship student Ray Shahlori have used to code up the solution procedure in R. I will show some examples of solutions.
http://www.stat.auckland.ac.nz/showperson?firstname=Rolf&surname=Turner
A Bayesian paradigm for finite population survey design and analysis
Speaker: Professor Murray Aitkin
Affiliation: Dept. of Mathematics and Statistics, U. Melbourne
When: Friday, 2 March 2012, 11:00 am to 12:00 pm
Where: ECE briefing room 303.257, Science Centre
A full Bayesian analysis for finite population survey sampling was described by Hartley and Rao in 1968 Biometrika, further developed by Ericson in 1969 JASA, and a non-informative prior version given by Rubin in 1981 as the Bayesian bootstrap.
This talk illustrates the advantages of this approach relative to current repeated sampling approaches, and describes extensions to handle clustering and stratification with different sampling fractions, including PPS sampling.
An illustration is given with a Labor Force survey example.
http://www.ms.unimelb.edu.au/~maitkin/
How big are the real mortality reductions produced by cancer screening? Why do so many trials report only 20%?
Speaker: Professor Jim Hanley
Affiliation: Department of Epidemiology, Biostatistics, and Occupational Health, McGill University
When: Monday, 20 February 2012, 11:00 am to 12:00 pm
Where: ECE Seminar Room 303.257, Science Centre
Influential reports on the reductions produced by screening for cancers of the prostate, colon and lung have appeared recently. The reported reductions in these randomized trials have been modest, and smaller than expected. But even more surprisingly, all three figures are very similar. I explain why these figures are underestimates and why the seemingly-universal 20% reduction is an artifact of the prevailing data-analysis methods and stopping rules. A different approach to the analysis of data from cancer screening trials is called for.
http://www.medicine.mcgill.ca/epidemiology/hanley/
On Akaike and likelihood cross-validation criteria for model selection
Speaker: Dr. Benoit Liquet
Affiliation: INSERM, Victor Segalen University, Bordeaux 2
When: Thursday, 16 February 2012, 4:00 pm to 5:00 pm
Where: ECE Seminar Room 303.257, Science Centre
The talk discusses Akaike and likelihood crossvalidation criteria for model/estimator choice. After a presentation of the main concept on model selection, we will focus on the choice of estimators in non-standard cases. First, we study two examples arising when we wish to assess the quality of estimators on a particular set of information, while the estimators may use a larger set of information. The first example occurs when we construct a model for an event which happens if a continuous variable is above a certain threshold. We can compare estimators based on the observation of only the event or on the whole continuous variable. The other example is that of predicting survival based on survival information only, or using in addition information on patient's disease. We develop modified AIC and LCV criteria to compare estimators in this non-standard situation. Second, we study the choice of estimators in prognostic studies. Estimators for a clinical event may use repeated measurements of markers in addition to fixed covariates. These measurements can be linked to the clinical event by joint modelling involving latent structures. When the objective is to choose between different estimators based on joint models for prediction, the conventional Akaike information criterion (AIC) is not well adapted and decision should be based on predictive accuracy. We define an adapted risk function called expected prognostic cross entropy (EPCE) and further modify it for right-censored observations. The risk functions can be estimated by leave-one-out cross validation, for which we give approximate formulas and asymptotic distributions.